Learn to define, analyze, and estimate causal effects using modern statistical methods for observational data.
Learn to define, analyze, and estimate causal effects using modern statistical methods for observational data.
This intermediate-level course introduces learners to the fundamental concepts and methods of causal inference. Over five weeks, you'll explore how causal effects are defined using potential outcomes, learn to express assumptions with causal graphs, and implement popular statistical methods such as matching, instrumental variables, and inverse probability of treatment weighting. The course emphasizes practical application, with opportunities to use R for data analysis. By the end, you'll be equipped to identify and estimate causal effects in various fields of study, moving beyond mere correlation to understand true causation.
4.7
(554 ratings)
42,529 already enrolled
Instructors:
English
پښتو, বাংলা, اردو, 2 more
What you'll learn
Define causal effects using potential outcomes
Distinguish between association and causation
Express assumptions using causal graphs (DAGs)
Implement matching and propensity score methods
Apply inverse probability of treatment weighting (IPTW)
Understand and use instrumental variables
Skills you'll gain
This course includes:
10.5 Hours PreRecorded video
16 quizzes
Access on Mobile, Tablet, Desktop
FullTime access
Shareable certificate
Closed caption
Get a Completion Certificate
Share your certificate with prospective employers and your professional network on LinkedIn.
Created by
Provided by
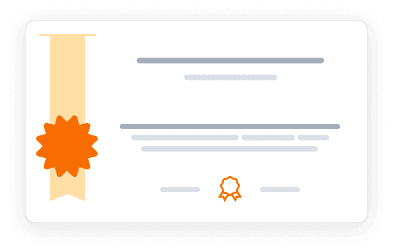
Top companies offer this course to their employees
Top companies provide this course to enhance their employees' skills, ensuring they excel in handling complex projects and drive organizational success.
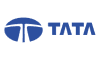
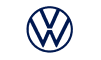
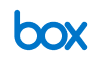
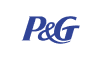
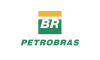
There are 5 modules in this course
This comprehensive course on causal inference equips learners with essential skills to analyze and interpret causal relationships in observational data. Through a structured curriculum, students explore key concepts such as potential outcomes, directed acyclic graphs, and various statistical methods for estimating causal effects. The course emphasizes both theoretical understanding and practical application, with hands-on exercises using R. Topics covered include confounding, matching techniques, propensity scores, inverse probability weighting, and instrumental variables. By the end of the course, learners will be able to design and implement causal inference analyses, critically evaluate causal claims, and apply these methods across various disciplines.
Welcome and Introduction to Causal Effects
Module 1 · 3 Hours to complete
Confounding and Directed Acyclic Graphs (DAGs)
Module 2 · 2 Hours to complete
Matching and Propensity Scores
Module 3 · 5 Hours to complete
Inverse Probability of Treatment Weighting (IPTW)
Module 4 · 3 Hours to complete
Instrumental Variables Methods
Module 5 · 3 Hours to complete
Fee Structure
Payment options
Financial Aid
Instructor
Expert in Biostatistics and Causal Inference
Dr. Jason A. Roy is a Professor and Chair of the Department of Biostatistics and Epidemiology at Rutgers University, as well as an Adjunct Professor at the University of Pennsylvania. He received his PhD in Biostatistics from the University of Michigan in 2000. Dr. Roy's research primarily focuses on causal inference, missing data, Bayesian methods, and pharmacoepidemiology. He is also the Co-Director of the Center for Causal Inference at Penn, where he contributes to advancing methodologies that improve understanding of causal relationships in health data.Dr. Roy teaches courses such as "A Crash Course in Causality: Inferring Causal Effects from Observational Data," which equips students with essential skills to analyze and interpret complex health data. His significant contributions to the field include published works on Bayesian nonparametric inference and marginal structural models, showcasing his expertise in statistical methods applied to real-world health issues.
Testimonials
Testimonials and success stories are a testament to the quality of this program and its impact on your career and learning journey. Be the first to help others make an informed decision by sharing your review of the course.
Frequently asked questions
Below are some of the most commonly asked questions about this course. We aim to provide clear and concise answers to help you better understand the course content, structure, and any other relevant information. If you have any additional questions or if your question is not listed here, please don't hesitate to reach out to our support team for further assistance.