Master statistical analysis of binary outcomes using logistic regression for healthcare data. Learn prediction and model assessment.
Master statistical analysis of binary outcomes using logistic regression for healthcare data. Learn prediction and model assessment.
This course cannot be purchased separately - to access the complete learning experience, graded assignments, and earn certificates, you'll need to enroll in the full Data Science for Health Research Specialization program. You can audit this specific course for free to explore the content, which includes access to course materials and lectures. This allows you to learn at your own pace without any financial commitment.
Instructors:
English
What you'll learn
Understand binary outcomes and calculate prevalence, risk ratios, and odds ratios
Use logistic regression for estimating associations between predictors and outcomes
Develop predictive models for healthcare applications
Assess model quality using calibration and discrimination metrics
Apply statistical analysis techniques to real healthcare data
Skills you'll gain
This course includes:
4.6 Hours PreRecorded video
7 quizzes
Access on Mobile, Tablet, Desktop
FullTime access
Shareable certificate
Get a Completion Certificate
Share your certificate with prospective employers and your professional network on LinkedIn.
Created by
Provided by
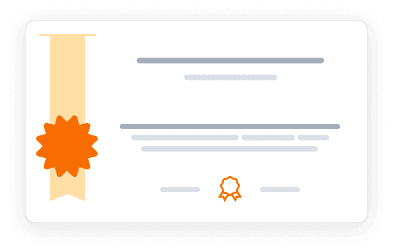
Top companies offer this course to their employees
Top companies provide this course to enhance their employees' skills, ensuring they excel in handling complex projects and drive organizational success.
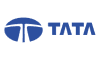
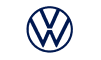
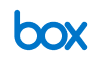
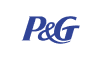
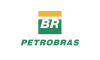
There are 3 modules in this course
This comprehensive course focuses on analyzing binary outcomes in healthcare data using logistic regression. Students learn fundamental statistical concepts, from basic two-group comparisons to advanced predictive modeling. The curriculum covers essential topics including prevalence calculation, risk ratios, odds ratios interpretation, and model assessment techniques. Through a combination of theoretical understanding and practical R programming exercises, learners develop skills in fitting logistic regression models, making predictions, and evaluating model performance using metrics like ROC curves and AUC.
Simple Comparisons of Binary Outcomes
Module 1 · 4 Hours to complete
Introducing Logistic Regression
Module 2 · 3 Hours to complete
Assessing the Predictive Accuracy of Logistic Regression Models
Module 3 · 3 Hours to complete
Fee Structure
Instructors
Leader in Epidemiology and Public Health Research
Bhramar Mukherjee holds the John D. Kalbfleisch Distinguished Professorship and serves as a Professor of Epidemiology and Global Public Health at the University of Michigan School of Public Health. Additionally, she is the Associate Director for Quantitative Data Sciences at the University of Michigan Rogel Cancer Center. Her research focuses on statistical methods for analyzing electronic health records, gene-environment interactions, Bayesian methods, shrinkage estimation, and high-dimensional exposure data analysis. During the COVID-19 pandemic, Bhramar and her team actively modeled the trajectory of the SARS-CoV-2 virus in India, gaining recognition in major media outlets such as Reuters, BBC, NPR, The New York Times, The Wall Street Journal, Der Spiegel, Australian National Radio, and The Times of India. With over 360 co-authored articles in statistics, biostatistics, medicine, and public health, she is also the founding director of the University of Michigan's Summer Institute on Big Data. Bhramar is a fellow of both the American Statistical Association and the American Association for the Advancement of Science, and she has received numerous awards for her scholarship, service, and teaching, including the Gertrude Cox Award from the Washington Statistical Society in 2016 and the L. Adrienne Cupples Award from Boston University in 2020. In 2021, she was honored with the Distinguished Woman Scholar Award from Purdue University, the Janet L. Norwood Award from the University of Alabama at Birmingham, and the Sarah Goddard Power Award from the University of Michigan Academic Women’s Caucus. Most notably, she was elected as a member of the US National Academy of Medicine in 2022 and received the Karl E. Peace Award for her statistical contributions toward societal betterment from the American Statistical Association in 2023.
Associate Professor
Phil is a faculty member and biostatistician in the Department of Biostatistics at the University of Michigan, Ann Arbor, USA. He studied Mathematics and Political Science at Calvin College (now Calvin University) in Grand Rapids, MI and received his MS and PhD in Biostatistics in 2009 and 2012, respectively, from the University of Michigan. He loves teaching biostatistics and R and collaborating with physicians and scientists, especially in research related to extracorporeal membrane oxygenation (ECMO) and oncology. When he’s not coding or doing statistics, you will often find him playing board games with his family and friends.
Testimonials
Testimonials and success stories are a testament to the quality of this program and its impact on your career and learning journey. Be the first to help others make an informed decision by sharing your review of the course.
Frequently asked questions
Below are some of the most commonly asked questions about this course. We aim to provide clear and concise answers to help you better understand the course content, structure, and any other relevant information. If you have any additional questions or if your question is not listed here, please don't hesitate to reach out to our support team for further assistance.