Master explainable AI in healthcare with hands-on implementation of LIME, SHAP, and attention mechanisms for deep learning model interpretability.
Master explainable AI in healthcare with hands-on implementation of LIME, SHAP, and attention mechanisms for deep learning model interpretability.
This course cannot be purchased separately - to access the complete learning experience, graded assignments, and earn certificates, you'll need to enroll in the full Informed Clinical Decision Making using Deep Learning Specialization program. You can audit this specific course for free to explore the content, which includes access to course materials and lectures. This allows you to learn at your own pace without any financial commitment.
4.6
(15 ratings)
1,623 already enrolled
Instructors:
English
What you'll learn
Program global explainability methods for time-series classification
Implement local explainability methods like CAM and GRAD-CAM
Master axiomatic attributions for deep learning networks
Incorporate and visualize attention mechanisms in RNNs
Understand the difference between interpretability and explainability
Skills you'll gain
This course includes:
3.13 Hours PreRecorded video
5 assignments
Access on Mobile, Tablet, Desktop
FullTime access
Shareable certificate
Get a Completion Certificate
Share your certificate with prospective employers and your professional network on LinkedIn.
Created by
Provided by
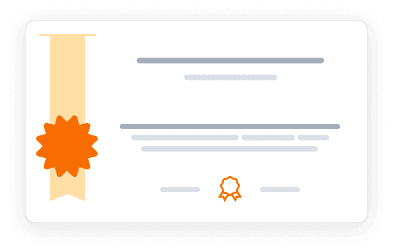
Top companies offer this course to their employees
Top companies provide this course to enhance their employees' skills, ensuring they excel in handling complex projects and drive organizational success.
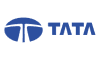
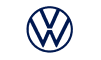
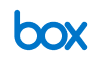
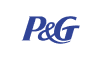
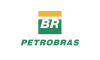
There are 4 modules in this course
This comprehensive course explores explainable deep learning models in healthcare applications. Students learn to implement and understand both global and local explainability methods, including Permutation Feature Importance, LIME, SHAP, and Class-Activation Mapping. The curriculum covers advanced concepts like axiomatic attributions and attention mechanisms in Recurrent Neural Networks, with practical applications in time-series classification. Through hands-on projects and real-world healthcare examples, learners develop skills in creating transparent and interpretable AI models.
Interpretable vs Explainable ML Models
Module 1 · 9 Hours to complete
Local Explainability Methods
Module 2 · 7 Hours to complete
Gradient-weighted CAM and Integrated Gradients
Module 3 · 7 Hours to complete
Attention mechanisms in Deep Learning
Module 4 · 5 Hours to complete
Fee Structure
Instructor
Leading Expert in Medical Image Computing and Healthcare Technology
Dr. Fani Deligianni serves as a Senior Lecturer/Associate Professor at the University of Glasgow's School of Computing Science, where she leads the Computing Technologies for Healthcare Theme. Her extensive educational background includes a PhD in Medical Image Computing from Imperial College London, two master's degrees (MSc in Advanced Computing from Imperial College London and MSc in Neuroscience from University College London), and a MEng in Electrical and Computer Engineering from Aristotle University, Greece. As a Fellow of the Higher Education Academy, she has demonstrated exceptional commitment to academic excellence and research innovation. Her research has garnered significant attention with over 50 peer-reviewed publications in prestigious venues, achieving an h-index of 22 and 2,719 citations. Her expertise in healthcare technology has attracted over £700,000 in competitive funding from organizations including EPSRC, MRC, and the Royal Society. Dr. Deligianni's research interests span medical image computing, machine learning in healthcare, human motion analysis, and brain connectivity, making her a key figure in advancing healthcare technologies through computational methods.
Testimonials
Testimonials and success stories are a testament to the quality of this program and its impact on your career and learning journey. Be the first to help others make an informed decision by sharing your review of the course.
4.6 course rating
15 ratings
Frequently asked questions
Below are some of the most commonly asked questions about this course. We aim to provide clear and concise answers to help you better understand the course content, structure, and any other relevant information. If you have any additional questions or if your question is not listed here, please don't hesitate to reach out to our support team for further assistance.