Master deep learning algorithms for text classification in marketing data. Learn Python implementation for automated categorization tasks.
Master deep learning algorithms for text classification in marketing data. Learn Python implementation for automated categorization tasks.
This course cannot be purchased separately - to access the complete learning experience, graded assignments, and earn certificates, you'll need to enroll in the full Text Marketing Analytics Specialization program. You can audit this specific course for free to explore the content, which includes access to course materials and lectures. This allows you to learn at your own pace without any financial commitment.
3.1
(14 ratings)
1,739 already enrolled
Instructors:
English
What you'll learn
Understand text classification and supervised machine learning concepts
Apply text classification techniques to marketing datasets
Develop practical skills through structured homework assignments
Train and evaluate text classification models
Implement deep learning solutions for marketing problems
Skills you'll gain
This course includes:
3.03 Hours PreRecorded video
3 quizzes
Access on Mobile, Tablet, Desktop
FullTime access
Shareable certificate
Get a Completion Certificate
Share your certificate with prospective employers and your professional network on LinkedIn.
Created by
Provided by
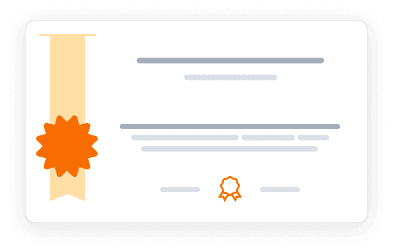
Top companies offer this course to their employees
Top companies provide this course to enhance their employees' skills, ensuring they excel in handling complex projects and drive organizational success.
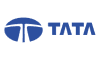
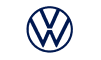
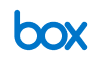
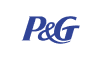
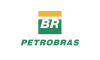
There are 4 modules in this course
This comprehensive course focuses on applying supervised deep learning techniques to marketing data classification tasks. Students learn the fundamentals of text classification, supervised machine learning workflows, and neural networks through hands-on Python tutorials. The curriculum covers data preparation, model training, performance evaluation, and real-world marketing applications. Through structured homework and a major project, students gain practical experience in implementing and optimizing text classification models for marketing analytics.
The Supervised Machine Learning Workflow
Module 1 · 7 Hours to complete
Neural Networks and Deep Learning
Module 2 · 1 Hours to complete
Getting Started with Google Colab and Deep Learning
Module 3 · 1 Hours to complete
Linear Models and Classification Metrics
Module 4 · 1 Hours to complete
Fee Structure
Instructors
Associate Professor
Dr. Chris J. Vargo is an Associate Professor at the University of Colorado Boulder, specializing in data analytics and digital advertising. His research integrates computer science techniques to analyze social media data through the lenses of communication, psychology, and political science. With expertise in data mining, machine learning, and predictive analytics, Dr. Vargo employs advanced methodologies such as network analysis and information retrieval to investigate contemporary media landscapes. His goal is to enhance the quantitative analytical skills of students, preparing them for the demands of the industry.In the classroom, Dr. Vargo teaches various courses related to advertising analytics at both undergraduate and graduate levels, including "Introduction to Digital Advertising" and "Programmatic Advertising." He also directs the CMCI/Leeds Marketing and Business Analytics partnership, fostering collaboration between departments. His scholarly contributions have been published in notable journals such as the Journal of Communication and New Media & Society. Additionally, he serves as the Editor of The Agenda Setting Journal, focusing on agenda-setting theory in new media contexts. With a robust academic background that includes a Ph.D. from The University of North Carolina at Chapel Hill and practical experience in public relations and digital marketing, Chris J. Vargo is dedicated to advancing research and education in the rapidly evolving field of digital advertising.
Senior Engineer
Scott Bradley is an Instructor at the University of Colorado Boulder, specializing in marketing analytics and data science. He teaches several courses, including "Network Analysis for Marketing Analytics," "Supervised Text Classification for Marketing Analytics," and "Unsupervised Text Classification for Marketing Analytics." His expertise lies in applying advanced analytical techniques to enhance marketing strategies and improve decision-making processes within organizations.With a strong background in data analysis and machine learning, Scott combines theoretical knowledge with practical applications to equip students with the skills necessary for success in the rapidly evolving field of data science. His courses focus on the intersection of data analytics and marketing, providing students with valuable insights into how data can drive effective marketing strategies. Through his teaching, Scott Bradley plays a crucial role in preparing the next generation of data-driven marketers at CU Boulder.
Testimonials
Testimonials and success stories are a testament to the quality of this program and its impact on your career and learning journey. Be the first to help others make an informed decision by sharing your review of the course.
3.1 course rating
14 ratings
Frequently asked questions
Below are some of the most commonly asked questions about this course. We aim to provide clear and concise answers to help you better understand the course content, structure, and any other relevant information. If you have any additional questions or if your question is not listed here, please don't hesitate to reach out to our support team for further assistance.