Master core data mining techniques including pattern analysis, classification, clustering, and outlier detection.
Master core data mining techniques including pattern analysis, classification, clustering, and outlier detection.
This course cannot be purchased separately - to access the complete learning experience, graded assignments, and earn certificates, you'll need to enroll in the full Data Mining Foundations and Practice Specialization program. You can audit this specific course for free to explore the content, which includes access to course materials and lectures. This allows you to learn at your own pace without any financial commitment.
3.7
(21 ratings)
5,965 already enrolled
Instructors:
English
What you'll learn
Master core data mining techniques and algorithms
Implement pattern analysis and association rules
Apply various classification and clustering methods
Perform outlier and anomaly detection
Evaluate and compare different mining approaches
Analyze complex data types and structures
Skills you'll gain
This course includes:
8.2 Hours PreRecorded video
3 programming assignments
Access on Mobile, Tablet, Desktop
FullTime access
Shareable certificate
Closed caption
Get a Completion Certificate
Share your certificate with prospective employers and your professional network on LinkedIn.
Created by
Provided by
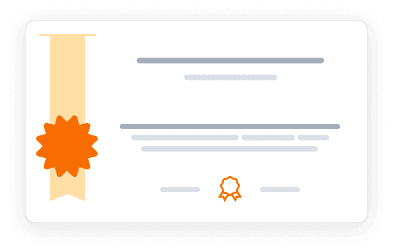
Top companies offer this course to their employees
Top companies provide this course to enhance their employees' skills, ensuring they excel in handling complex projects and drive organizational success.
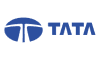
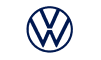
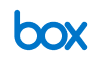
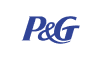
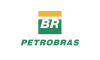
There are 4 modules in this course
This comprehensive course explores advanced data mining techniques and their practical applications. Students learn essential methods including frequent pattern analysis, classification algorithms, clustering approaches, and outlier detection. The curriculum covers both theoretical foundations and hands-on implementation of various techniques, from basic algorithms like Apriori to advanced topics like neural networks and ensemble methods. Special emphasis is placed on evaluating and selecting appropriate methods for different data mining tasks.
Frequent Pattern Analysis
Module 1 · 7 Hours to complete
Classification
Module 2 · 6 Hours to complete
Clustering
Module 3 · 5 Hours to complete
Outlier Analysis
Module 4 · 4 Hours to complete
Fee Structure
Instructor
Professor
Qin (Christine) Lv is a Professor in the Department of Computer Science at the University of Colorado Boulder, where she specializes in full-stack data analytics, integrating systems, algorithms, and applications for effective data analytics in ubiquitous computing and scientific discovery. She earned her B.E. with honors from Tsinghua University and both an M.A. and Ph.D. from Princeton University, where she was advised by Professor Kai Li. Dr. Lv's research interests include mobile and wearable computing, social networks, spatial-temporal data, anomaly detection, similarity search, and recommender systems, with interdisciplinary applications in environmental research and renewable energy. She has received several prestigious awards, including the SenSys 2018 Best Paper Runner-up Award and the 2017 Google Faculty Research Award, and has held significant roles in professional activities, such as General Co-chair for UbiComp 2021. Dr. Lv can be reached at her office in Engineering Center ECCR 1B24 or via email.
Testimonials
Testimonials and success stories are a testament to the quality of this program and its impact on your career and learning journey. Be the first to help others make an informed decision by sharing your review of the course.
Frequently asked questions
Below are some of the most commonly asked questions about this course. We aim to provide clear and concise answers to help you better understand the course content, structure, and any other relevant information. If you have any additional questions or if your question is not listed here, please don't hesitate to reach out to our support team for further assistance.