Master essential probability concepts and discrete random variables for data science and information analysis careers.
Master essential probability concepts and discrete random variables for data science and information analysis careers.
This introductory course provides a comprehensive foundation in mathematical probability, focusing on fundamental concepts and discrete random variable analysis. Designed for aspiring data scientists and actuarial professionals, the course covers essential probability theories, Bayes' theorem, probability distributions, and expected values. Students learn through practical problems and examples, exploring various distribution models including Bernoulli, Binomial, Geometric, and Poisson distributions. The curriculum bridges theoretical concepts with practical applications, preparing learners for advanced studies in data science and statistical analysis. Supported by the National Science Foundation's Center for Science of Information, this course serves as a crucial stepping stone for careers in information and data science.
Instructors:
English
English
What you'll learn
Master basic probability concepts and fundamental rules
Apply probability models to solve practical problems
Understand discrete random variables and their distributions
Analyze joint distributions and expected values
Implement Bayes theorem in probability calculations
Work with various probability distribution models
Skills you'll gain
This course includes:
PreRecorded video
Graded assignments, exams
Access on Mobile, Tablet, Desktop
Limited Access access
Shareable certificate
Closed caption
Get a Completion Certificate
Share your certificate with prospective employers and your professional network on LinkedIn.
Created by
Provided by
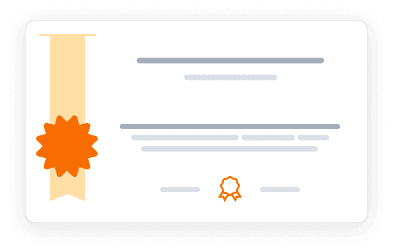
Top companies offer this course to their employees
Top companies provide this course to enhance their employees' skills, ensuring they excel in handling complex projects and drive organizational success.
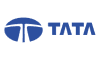
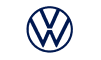
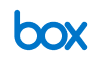
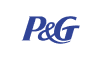
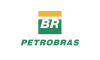
There are 6 modules in this course
This comprehensive course introduces fundamental probability concepts essential for data science and statistical analysis. The curriculum progresses through six units, covering sample spaces, probability rules, conditional probability, random variables, expected values, and various discrete probability distribution models. Students learn to apply probability concepts to practical problems, understand different distribution types, and develop a strong foundation in statistical theory. The course emphasizes both theoretical understanding and practical application, preparing students for advanced studies in data science and actuarial science.
Sample Space and Probability
Module 1
Conditional Probability and Independence
Module 2
Random Variables Fundamentals
Module 3
Expected Values
Module 4
Basic Discrete Distributions
Module 5
Advanced Discrete Distributions
Module 6
Instructor
Distinguished Data Science Pioneer Leading Innovation at Purdue University
Mark Daniel Ward serves as a Professor of Statistics at Purdue University, holding courtesy appointments in Agricultural & Biological Engineering, Computer Science, Mathematics, and Public Health. As Executive Director of The Data Mine, he has revolutionized data science education while maintaining an impressive research portfolio in probabilistic and combinatorial analysis of algorithms and data structures. His academic journey includes a B.S. from Denison University in Mathematics and Computer Science, an M.S. from the University of Wisconsin-Madison in Applied Mathematical Sciences, and a Ph.D. from Purdue University in Mathematics with Specialization in Computational Science. His excellence in both teaching and research has earned him numerous accolades, including Fellow of the American Statistical Association, the Focus Award, and membership in the International Statistical Institute. His research interests span across analytic combinatorics, applied probability, data compression, game theory, and information theory. Beyond his research, he has demonstrated exceptional leadership in education, winning multiple teaching awards including the College of Science Undergraduate Advising Award and being named a Fellow of the Purdue University Teaching Academy. His impact extends beyond traditional academics as he directs The Data Mine initiative, fostering large-scale computational research and data science education at Purdue
Testimonials
Testimonials and success stories are a testament to the quality of this program and its impact on your career and learning journey. Be the first to help others make an informed decision by sharing your review of the course.
Frequently asked questions
Below are some of the most commonly asked questions about this course. We aim to provide clear and concise answers to help you better understand the course content, structure, and any other relevant information. If you have any additional questions or if your question is not listed here, please don't hesitate to reach out to our support team for further assistance.