Master medical ML through practical healthcare projects: build disease prediction models, analyze patient data, and deploy clinical AI solutions.
Master medical ML through practical healthcare projects: build disease prediction models, analyze patient data, and deploy clinical AI solutions.
Explore the cutting-edge intersection of machine learning and healthcare in this comprehensive course from MITx. Over 15 weeks, dive deep into the application of ML techniques for risk stratification, disease progression modeling, clinical workflows, and precision medicine. Gain hands-on experience with Python projects using real healthcare data, covering topics from deep learning on medical imaging to natural language processing of clinical texts. This course balances theoretical foundations with practical considerations, addressing crucial aspects like model interpretability, fairness, and regulatory compliance in healthcare AI. Ideal for students and professionals with a strong background in machine learning, looking to specialize in healthcare applications.
Instructors:
English
English
What you'll learn
Apply machine learning methods for risk stratification and disease diagnosis
Develop models for disease progression and precision medicine
Implement deep learning techniques for medical imaging analysis
Apply natural language processing to clinical text data
Design interpretable and fair machine learning models for healthcare
Analyze physiological time-series data using ML techniques
Skills you'll gain
This course includes:
Live video
Programming projects, Homework assignments
Access on Mobile, Tablet, Desktop
Limited Access access
Shareable certificate
Closed caption
Get a Completion Certificate
Share your certificate with prospective employers and your professional network on LinkedIn.
Created by
Provided by
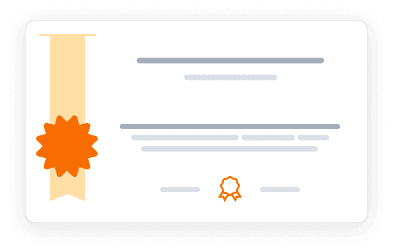
Top companies offer this course to their employees
Top companies provide this course to enhance their employees' skills, ensuring they excel in handling complex projects and drive organizational success.
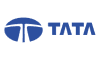
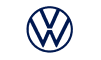
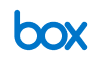
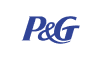
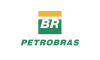
There are 6 modules in this course
This advanced course explores the application of machine learning techniques in healthcare, covering a wide range of topics from risk stratification and diagnosis to disease progression modeling and precision medicine. Students will work with various types of clinical data, including physiological time-series, clinical text, and medical images. The curriculum emphasizes both theoretical foundations and practical implementations, with a focus on the unique challenges of healthcare data. Key areas include supervised prediction models, clinical natural language processing, interpretability analysis, and causal inference. The course also addresses critical human factors in healthcare AI, such as model transparency, fairness, and regulatory compliance. Through hands-on Python projects and guest lectures by clinicians, students will gain a comprehensive understanding of translating machine learning into clinical practice.
Overview of Clinical Care & Data
Module 1 · 2 Weeks to complete
ML for Risk Stratification & Diagnosis
Module 2 · 3 Weeks to complete
ML with clinical text, imaging, and physiological data
Module 3 · 2 Weeks to complete
Understanding disease and its progression
Module 4 · 2 Weeks to complete
Human Factors
Module 5 · 3 Weeks to complete
Causal Inference & Reinforcement Learning
Module 6 · 3 Weeks to complete
Fee Structure
Instructors
Healthcare Data Scientist Advancing Medical AI Applications
Hagai Rossman serves as a researcher at Pheno.AI after completing his PhD in Computer Science at the Weizmann Institute of Science's Segal Lab. His groundbreaking research focuses on medical data science, epidemiology, and machine learning applications in healthcare. His significant contributions include leading studies on COVID-19 dynamics after Israel's national immunization program and developing frameworks for identifying regional disease outbreaks. His work has appeared in prestigious journals including Nature Medicine, Nature Communications, and The Lancet Digital Health, with his publications receiving over 1,700 citations. Through his research on healthcare data analysis, disease prediction models, and national health surveillance systems, he continues to advance the intersection of artificial intelligence and medical applications while collaborating with physicians to improve healthcare outcomes.
Medical Informatics Researcher Advancing COVID-19 Analytics
Zachary H. Strasser serves as a National Library of Medicine postdoctoral fellow at Harvard Medical School and Massachusetts General Hospital's Laboratory of Computer Science, while maintaining clinical practice as an internist. His research combines medical informatics with clinical care, focusing on developing novel phenotyping tools and studying COVID-19 epidemiology. His recent work includes leading a groundbreaking study analyzing post-acute sequelae of COVID-19 (PASC) across three major healthcare systems, utilizing advanced machine learning techniques to identify and validate symptom patterns1
Testimonials
Testimonials and success stories are a testament to the quality of this program and its impact on your career and learning journey. Be the first to help others make an informed decision by sharing your review of the course.
Frequently asked questions
Below are some of the most commonly asked questions about this course. We aim to provide clear and concise answers to help you better understand the course content, structure, and any other relevant information. If you have any additional questions or if your question is not listed here, please don't hesitate to reach out to our support team for further assistance.