Master essential linear algebra concepts for machine learning, from vectors and matrices to eigenvalues and PageRank algorithm implementation.
Master essential linear algebra concepts for machine learning, from vectors and matrices to eigenvalues and PageRank algorithm implementation.
This course cannot be purchased separately - to access the complete learning experience, graded assignments, and earn certificates, you'll need to enroll in the full Mathematics for Machine Learning Specialization program. You can audit this specific course for free to explore the content, which includes access to course materials and lectures. This allows you to learn at your own pace without any financial commitment.
4.7
(12,095 ratings)
3,91,944 already enrolled
Instructors:
English
বাংলা, اردو, Tiếng Việt, 2 more
What you'll learn
Understand and manipulate vectors and matrices in linear algebra
Master eigenvalues and eigenvectors calculation and applications
Implement linear transformations and basis changes
Apply linear algebra concepts to real-world data problems
Develop practical coding skills for mathematical operations
Skills you'll gain
This course includes:
3.7 Hours PreRecorded video
15 quizzes, 4 programming assignments
Access on Mobile, Tablet, Desktop
FullTime access
Shareable certificate
Get a Completion Certificate
Share your certificate with prospective employers and your professional network on LinkedIn.
Created by
Provided by
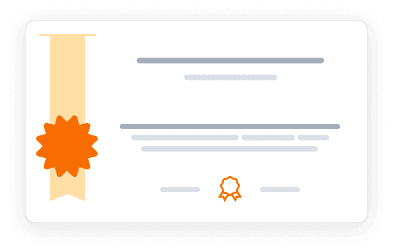
Top companies offer this course to their employees
Top companies provide this course to enhance their employees' skills, ensuring they excel in handling complex projects and drive organizational success.
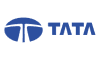
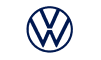
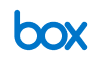
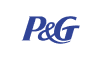
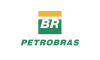
There are 5 modules in this course
This comprehensive course explores linear algebra fundamentals essential for machine learning applications. Students learn about vectors, matrices, eigenvalues, and eigenvectors through practical examples and hands-on programming exercises. The curriculum progresses from basic vector operations to advanced concepts like the PageRank algorithm, combining theoretical understanding with practical implementation in Python. Special emphasis is placed on developing mathematical intuition rather than just computational skills.
Introduction to Linear Algebra and to Mathematics for Machine Learning
Module 1 · 2 Hours to complete
Vectors are objects that move around space
Module 2 · 1 Hours to complete
Matrices in Linear Algebra: Objects that operate on Vectors
Module 3 · 3 Hours to complete
Matrices make linear mappings
Module 4 · 6 Hours to complete
Eigenvalues and Eigenvectors: Application to Data Problems
Module 5 · 4 Hours to complete
Fee Structure
Instructors
Strategic Teaching Fellow in Design Engineering
Dr. Freddie Page serves as the Strategic Teaching Fellow in the Dyson School of Design Engineering at Imperial College London. He earned his MPhys from the University of Oxford in 2011 and completed his PhD in theoretical nanophotonics at Imperial College London in 2016. His research focuses on designing materials capable of slowing light to a complete stop and exploring the interactions of light with sheets of graphene far from thermal equilibrium.
Professor David Dye: Expert in Alloy Development and Materials Science
David Dye is a Professor of Metallurgy in the Department of Materials, specializing in the development of alloys for jet engines, nuclear applications, and caloric materials aimed at reducing fuel consumption and preventing in-service failures. His work involves advanced crystallography, utilizing techniques such as neutron and synchrotron X-ray diffraction and electron microscopy at the atomic scale. The large datasets generated by these techniques present complex data analysis challenges. David earned his PhD and undergraduate degrees from Cambridge University in 1997 and 2000, respectively, and joined Imperial College London in 2003. He also teaches introductory mathematics, with a focus on errors and data analysis, and has been recognized with student-led awards for his innovative teaching methods.
Testimonials
Testimonials and success stories are a testament to the quality of this program and its impact on your career and learning journey. Be the first to help others make an informed decision by sharing your review of the course.
Frequently asked questions
Below are some of the most commonly asked questions about this course. We aim to provide clear and concise answers to help you better understand the course content, structure, and any other relevant information. If you have any additional questions or if your question is not listed here, please don't hesitate to reach out to our support team for further assistance.