Learn to use graphical tools for causal inference in research design and data analysis with this Harvard course.
Learn to use graphical tools for causal inference in research design and data analysis with this Harvard course.
This course introduces causal diagrams as a revolutionary tool for understanding and analyzing causal relationships in research. It focuses on how to use intuitive pictures to improve study design and data analysis for causal inference. The curriculum covers the basics of causal directed acyclic graphs (DAGs) and their rules, application to common forms of bias, representation of time-varying treatments and treatment-confounder feedback, and the introduction of Single World Intervention Graphs (SWIGs). Learners will develop skills in translating expert knowledge into causal diagrams, identifying common biases, and using these diagrams to guide data analysis. The course includes both theoretical foundations and practical applications, featuring case studies from health and social sciences to demonstrate real-world usage of causal diagrams.
4.9
(17 ratings)
84,265 already enrolled
Instructors:
English
English
What you'll learn
Translate expert knowledge into causal diagrams
Construct causal diagrams under different assumptions
Use causal diagrams to identify common biases in research
Apply causal diagrams to guide data analysis
Understand the rules governing causal Directed Acyclic Graphs (DAGs)
Represent time-varying treatments and treatment-confounder feedback in causal diagrams
Skills you'll gain
This course includes:
PreRecorded video
Graded assignments, exams
Access on Mobile, Tablet, Desktop
Limited Access access
Shareable certificate
Closed caption
Get a Completion Certificate
Share your certificate with prospective employers and your professional network on LinkedIn.
Created by
Provided by
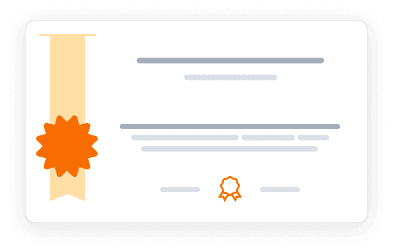
Top companies offer this course to their employees
Top companies provide this course to enhance their employees' skills, ensuring they excel in handling complex projects and drive organizational success.
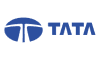
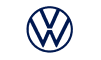
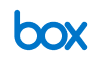
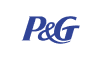
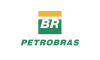
Module Description
This course provides a comprehensive introduction to causal diagrams and their applications in research. It is structured into two main parts. The first part consists of seven lessons covering the fundamentals of causal diagrams, including causal DAGs, their rules, and applications to various forms of bias. It also introduces more advanced concepts like time-varying treatments and SWIGs. The second part of the course focuses on case studies, demonstrating how causal diagrams are applied to real-world research questions in health and social sciences. Throughout the course, learners will develop skills in constructing and interpreting causal diagrams, using them to identify potential biases, and guiding data analysis for causal inference. The curriculum emphasizes both theoretical understanding and practical application, preparing learners to use these powerful tools in their own research.
Fee Structure
Instructor
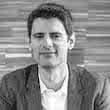
1 Course
Leading Causal Inference Methodologist Revolutionizing Public Health Research
Miguel Hernán, Kolokotrones Professor of Biostatistics and Epidemiology at Harvard T.H. Chan School of Public Health, has transformed epidemiological research through pioneering work in causal inference methodology. After earning his medical degree from Universidad Autónoma de Madrid and multiple graduate degrees from Harvard, including a DrPH in Epidemiology, he has built an extraordinary career combining methodological innovation with practical applications. As Director of the CAUSALab at Harvard, he leads groundbreaking research using health data and causal inference methods to improve treatment and prevention of diseases ranging from HIV to cancer. His free online course "Causal Diagrams" has reached over 80,000 learners, while his co-authored book "Causal Inference: What If" has become a cornerstone text in the field. His achievements include the 2022 Rousseeuw Prize for Statistics, election as Fellow of both the American Association for the Advancement of Science and American Statistical Association, and a MERIT Award from the National Institutes of Health. Through his roles at Harvard-MIT Health Sciences and Technology and as Methods Editor for Annals of Internal Medicine, he continues to advance causal inference methodology while mentoring the next generation of researchers.
Testimonials
Testimonials and success stories are a testament to the quality of this program and its impact on your career and learning journey. Be the first to help others make an informed decision by sharing your review of the course.
4.9 course rating
17 ratings
Frequently asked questions
Below are some of the most commonly asked questions about this course. We aim to provide clear and concise answers to help you better understand the course content, structure, and any other relevant information. If you have any additional questions or if your question is not listed here, please don't hesitate to reach out to our support team for further assistance.