This course is part of Programming for Python Data Science: Principles to Practice.
This course cannot be purchased separately - to access the complete learning experience, graded assignments, and earn certificates, you'll need to enroll in the full Programming for Python Data Science: Principles to Practice Specialization program. You can audit this specific course for free to explore the content, which includes access to course materials and lectures. This allows you to learn at your own pace without any financial commitment.
Instructors:
English
What you'll learn
Create professional visualizations for various types of data using matplotlib
Implement and evaluate K-Nearest Neighbors algorithms for classification
Apply regression techniques to analyze relationships between variables
Customize plots for effective data communication
Build predictive models from scratch in Python
Differentiate between prediction and inference in data science context
Skills you'll gain
This course includes:
2.07 Hours PreRecorded video
4 assignments
Access on Mobile, Tablet, Desktop
FullTime access
Shareable certificate
Get a Completion Certificate
Share your certificate with prospective employers and your professional network on LinkedIn.
Created by
Provided by
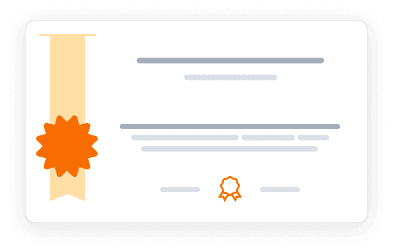
Top companies offer this course to their employees
Top companies provide this course to enhance their employees' skills, ensuring they excel in handling complex projects and drive organizational success.
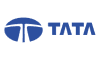
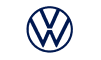
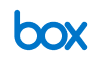
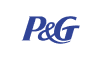
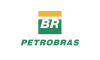
There are 4 modules in this course
This comprehensive course bridges the gap between programming and data science by teaching advanced visualization and modeling techniques in Python. Students begin with an extensive exploration of plotting using matplotlib, learning to create and customize a variety of visualizations from basic line, bar, and scatter plots to more complex histograms and heatmaps. The second module introduces predictive modeling with a focus on K-Nearest Neighbors (KNN) algorithms for both classification and regression tasks, including implementation from scratch and evaluation methodologies. The third module covers statistical modeling with linear regression for both prediction and inference, teaching students to implement regression models and interpret relationships between variables. The course culminates in a capstone project where students integrate all learned skills to recreate a famous Gapminder visualization by merging multiple datasets to illustrate the relationship between countries' income and greenhouse gas emissions. Throughout, students gain hands-on experience through interactive assignments, live coding demonstrations, and real-world data analyses, building a foundation for a career in data science.
Plotting
Module 1 · 11 Hours to complete
Prediction
Module 2 · 9 Hours to complete
Regression
Module 3 · 5 Hours to complete
Final Project
Module 4 · 4 Hours to complete
Fee Structure
Individual course purchase is not available - to enroll in this course with a certificate, you need to purchase the complete Professional Certificate Course. For enrollment and detailed fee structure, visit the following: Programming for Python Data Science: Principles to Practice
Instructors
Associate Professor of the Practice
Andrew Hilton is an Associate Professor of the Practice in the Department of Electrical and Computer Engineering at Duke University's Pratt School of Engineering, where he has been teaching since 2012. Before joining Duke, he worked as an advisory engineer at IBM. One of the key courses he teaches is ECE 551, an intensive introduction to programming designed to equip graduate students with no prior experience to master programming and tackle advanced courses. In 2015, Professor Hilton received the Klein Family Distinguished Teaching Award for his excellence in teaching. He holds a Ph.D. in Computer Science from the University of Pennsylvania.
Assistant Professor of the Practice at Duke University
Dr. Genevieve M. Lipp is an Assistant Professor of the Practice in the Electrical and Computer Engineering and Mechanical Engineering and Materials Science departments at Duke University. She teaches a variety of courses, including programming in C++, dynamics, control systems, and robotics. Dr. Lipp is passionate about integrating technology into education to enhance learning outcomes and has previously worked in the Center for Instructional Technology at Duke. She holds a Ph.D. in mechanical engineering, focusing on nonlinear dynamics, as well as a B.S.E. in mechanical engineering and a B.A. in German, both from Duke University. In addition to her teaching responsibilities, she serves as the Director of the Duke Engineering First Year Computing program, where she focuses on improving computing education within the engineering curriculum and fostering students' self-efficacy in their studies.
Testimonials
Testimonials and success stories are a testament to the quality of this program and its impact on your career and learning journey. Be the first to help others make an informed decision by sharing your review of the course.
Frequently asked questions
Below are some of the most commonly asked questions about this course. We aim to provide clear and concise answers to help you better understand the course content, structure, and any other relevant information. If you have any additional questions or if your question is not listed here, please don't hesitate to reach out to our support team for further assistance.